Transforming Business through Image Annotation Machine Learning
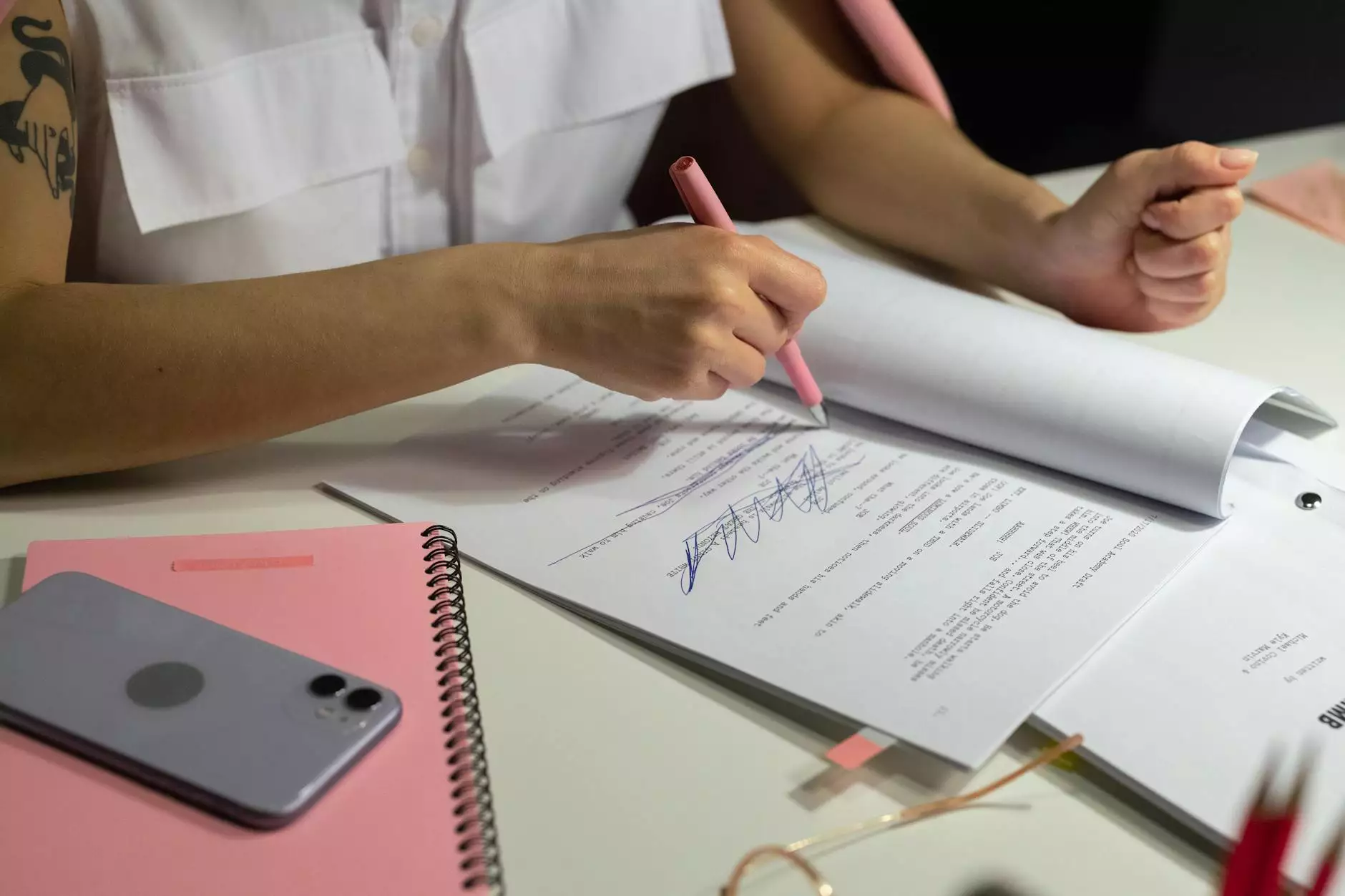
In today’s rapidly evolving technological landscape, image annotation machine learning plays a pivotal role in business operations across various sectors. As companies increasingly rely on data-driven decisions, the need for precise, accurate, and efficient data annotation tools and platforms has never been greater. This article delves deep into the definition, importance, and application of image annotation in machine learning, highlighting how organizations can leverage this technology for optimal results.
Understanding Image Annotation Machine Learning
Image annotation refers to the process of labeling images to provide context for machine learning models, enabling them to understand and interpret the visual content accurately. This is crucial for training algorithms in tasks such as object detection, image classification, and semantic segmentation. In the realm of machine learning, the quality of the data is paramount; without accurate annotations, even the most sophisticated algorithms can fail to deliver reliable results.
The Significance of Image Annotation in Machine Learning
Why is image annotation machine learning crucial for businesses? Here are several key reasons:
- Enhanced Accuracy: Properly annotated images lead to higher accuracy rates in predictions and classifications.
- Improved Decision Making: Businesses can make data-driven decisions by understanding visual data effectively.
- Increased Efficiency: Automating image annotation processes saves time and resources, allowing teams to focus on core business functions.
- Competitive Edge: Utilizing advanced annotation techniques can help businesses stay ahead of competitors by enhancing AI model performance.
Key Applications of Image Annotation in Business
Organizations utilize image annotation machine learning across diverse fields. Here are the primary applications:
1. Autonomous Vehicles
In the automotive industry, machine learning models require substantial amounts of labeled images to detect traffic signs, pedestrians, and other vehicles. Automated image annotation helps in training these systems to navigate safely and efficiently.
2. Healthcare
In medical imaging, the accuracy of image labels is critical. Annotated images enable algorithms to identify diseases, assist in diagnostics, and improve treatment plans, thus enhancing patient care.
3. Retail and E-commerce
Image annotation facilitates visual search capabilities in e-commerce platforms, allowing customers to find products through images rather than keywords. This improves user experience and can significantly increase conversion rates.
4. Agriculture
Farmers use machine learning to monitor crops through satellite and aerial imagery. Annotated images help in assessing crop health, predicting yields, and even detecting pest infestations.
5. Security and Surveillance
In security applications, image annotation aids in facial recognition and anomaly detection, enhancing surveillance systems' accuracy and efficiency.
Choosing the Right Data Annotation Tool
Selecting an appropriate data annotation tool is vital for businesses aiming to implement image annotation machine learning effectively. Here are some factors to consider:
- Usability: The interface should be user-friendly and intuitive.
- Accuracy: Ensure the tool provides high accuracy in annotations to improve machine learning model performance.
- Scalability: The tool should handle large datasets and scale as your business grows.
- Integration: It should seamlessly integrate with existing workflow systems and technologies.
- Support: Reliable support and training resources are essential for successful implementation.
The Process of Image Annotation
The process of annotating images involves several steps to ensure high-quality data for machine learning:
1. Image Selection
Select relevant images from your dataset that require annotation based on the task at hand.
2. Choosing Annotation Type
Decide on the appropriate annotation types:
- Bounding Boxes: Used for object detection to identify specific objects within images.
- Segmentation: Divides an image into segments for more nuanced analysis.
- Landmarks: Points in an image to indicate relevant features.
3. Annotation Tool Setup
Utilize the chosen annotation tool to begin the labeling process, ensuring adherence to guidelines for accuracy.
4. Quality Assurance
Implement quality checks and validations to maintain high standards in annotations, which directly impacts machine learning outcomes.
5. Data Integration
Once annotated, integrate the data back into your machine learning pipeline for training and model development.
The Future of Image Annotation in Machine Learning
As technology advances, the future of image annotation machine learning looks bright. We are witnessing several emerging trends:
1. Automation and AI-enhanced Annotation
Machine learning techniques are being employed to automate the annotation process, significantly reducing the time needed while improving quality.
2. Increased Use of Deep Learning
Deep learning models are set to enhance the capabilities of machine learning systems, allowing them to learn from vast amounts of annotated data more efficiently.
3. Real-time Annotation
Real-time image annotation capabilities are becoming increasingly available, providing dynamic and interactive systems capable of responding to input instantly.
Optimizing Your Workflow with Keylabs.ai
To stay competitive in the market, leveraging a robust data annotation platform like Keylabs.ai can make a considerable difference. Keylabs.ai specializes in providing state-of-the-art data annotation tools designed to maximize efficiency and accuracy. With features tailored for various industries, businesses can streamline their image annotation processes seamlessly.
Why Choose Keylabs.ai?
- High-Quality Annotations: Keylabs.ai focuses on delivering high-precision annotations that boost the performance of machine learning models.
- User-Friendly Interface: Designed for ease of use, teams can get started quickly without extensive training.
- Scalability: Whether you have a small dataset or a massive one, Keylabs.ai can handle it with ease.
- Expert Support: Access expert guidance and support to resolve issues quickly, ensuring smooth operations.
Conclusion
In conclusion, image annotation machine learning is not just a technical requirement; it is a foundation for building intelligent systems that can significantly enhance business efficiency and decision-making. As industries continue to demand higher accuracy and better insights, the role of advanced data annotation tools and platforms will only grow more critical. Partnering with providers like Keylabs.ai equips businesses with the necessary resources to accelerate their transformation and stay ahead in the competitive landscape.
Embrace the future today and see how image annotation can redefine your organization's capabilities!