The Essential Role of Bounding Boxes in Business and Technology
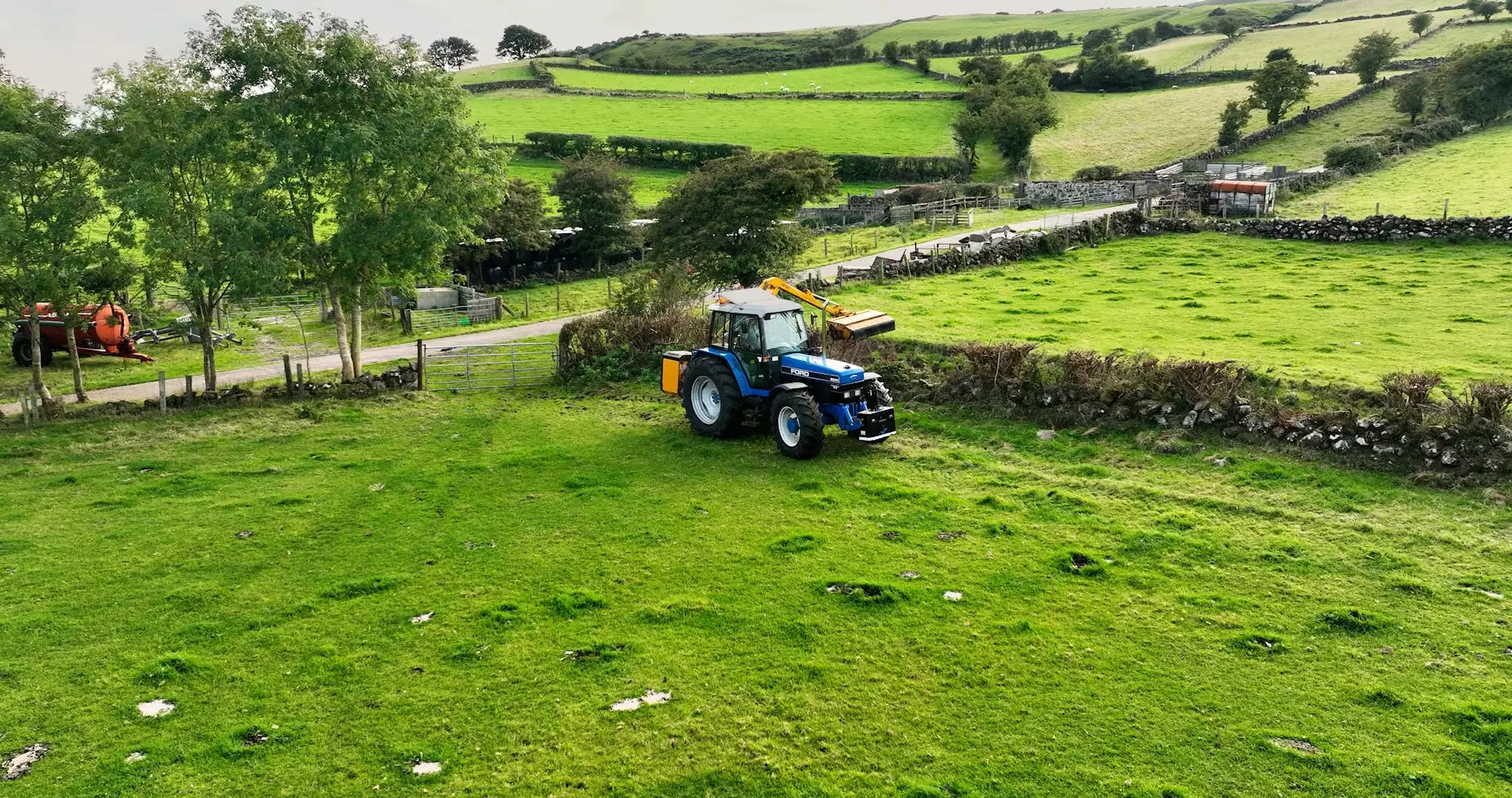
The term "bounding box" may evoke thoughts of geometry and mathematics, but in today’s technological landscape, it has become a cornerstone concept in various fields, particularly in computer graphics, computer vision, and image processing. Understanding how bounding boxes function can significantly enhance operational efficiencies in numerous business applications.
What is a Bounding Box?
A bounding box is defined as the smallest rectangle that can completely enclose a particular shape or object within a coordinate system. This fundamental concept serves as a tool to identify and manage objects in two-dimensional or three-dimensional spaces. Businesses leverage bounding boxes for various purposes, including but not limited to:
- Data Annotation - Annotating images and video frames using bounding boxes is essential for teaching machines to recognize objects accurately.
- Computer Vision Applications - Bounding boxes are widely used in machine learning models, particularly for training algorithms to identify, classify, and segment objects in images.
- Object Detection - Utilizing bounding boxes allows for effective object detection, an important aspect in surveillance, retail, and autonomous driving technologies.
How Bounding Boxes Enhance Data Annotation Tools
In the realm of data annotation, bounding boxes play an invaluable role. They serve as the groundwork upon which machine learning algorithms build their understanding of objects within images. Companies like Keylabs.ai have revolutionized data annotation processes by integrating sophisticated bounding box technology into their platforms. Here are several ways in which bounding boxes enhance data annotation:
1. Increased Accuracy
When annotating data, the precision of object localization is critical. Bounding boxes provide a clear demarcation of where objects exist in images, enabling more accurate annotations. This level of detail allows machine learning models to learn more effectively, improving overall performance.
2. Streamlined Workflow
Implementing bounding boxes within data annotation tools facilitates a streamlined workflow. Annotations can be performed quickly and efficiently, as users are provided with predictable guidelines for marking object boundaries. This not only saves time but also ensures consistency across datasets.
3. Scalability of Projects
As businesses expand their data needs, the ability to scale annotation projects becomes crucial. Bounding boxes allow teams to manage extensive datasets without compromising quality, thus making large projects feasible and more manageable.
The Impact of Bounding Boxes in Machine Learning
Bounding boxes play a pivotal role beyond mere annotation; they significantly influence the training of machine learning models. Here are some key impacts:
1. Enhanced Object Detection Algorithms
In the context of object detection, bounding boxes are instrumental. They provide the necessary training data for algorithms designed to identify and locate objects within images. As such, the efficacy of these algorithms is directly correlated with the quality of the bounding box annotations provided.
2. Improved Performance Metrics
Bounding boxes also enhance performance metrics such as precision and recall. Algorithms trained on accurately annotated data with bounding boxes perform better in real-world applications, leading to more reliable outcomes in various sectors, including healthcare, automotive, and retail.
3. Facilitating Real-time Applications
In applications such as autonomous vehicles or real-time video surveillance, the ability to quickly and accurately detect and classify objects is crucial. Bounding boxes enable these functionalities by providing instantaneous feedback regarding object locations, thereby facilitating critical decision-making processes.
Bounding Boxes in Business Applications
The practical application of bounding boxes transcends technical fields, extending into various business domains. Here are some notable applications:
1. Retail and Inventory Management
In the retail landscape, bounding boxes are utilized for effective inventory management by allowing retailers to recognize products on shelves through image recognition technology. This facilitates automated inventory checks and enhances the shopping experience. By establishing bounding boxes around products in images, algorithms can easily identify stock levels and product placements.
2. Surveillance and Security
In the realm of surveillance, the integration of bounding boxes is critical for identifying potential security threats. Advanced algorithms analyze video feeds, using bounding boxes to track moving objects. This enhances situational awareness for security personnel, ensuring rapid responses to potential incidents.
3. Healthcare Imaging
In healthcare, bounding boxes are crucial for analyzing medical images such as MRIs or CT scans. By assisting in the identification of tumors or anomalies within these images, bounding boxes help medical professionals render accurate diagnoses and improve patient outcomes.
Best Practices for Implementing Bounding Boxes in Data Annotation
- Consistency is Key - Ensure that all annotators are trained to apply bounding boxes uniformly to eliminate discrepancies in the dataset.
- Quality Control Measures - Regularly review annotated datasets for accuracy and consistency; this helps maintain high standards and improves model performance.
- Utilize Automated Tools - Leverage advanced annotation tools that streamline the bounding box creation process, making it more efficient and less error-prone.
- Feedback Loops - Establish feedback mechanisms where annotators can receive input on their annotations, fostering an environment of continuous improvement.
Future Trends in Bounding Box Technology
The landscape of technology is evolving rapidly, and the role of bounding boxes is no exception. Some emerging trends include:
1. Machine Learning Enhancements
As machine learning continues to advance, the algorithms will become even better at interpreting bounding boxes. Future iterations might enable automatic detection and creation of bounding boxes, further enhancing efficiency and accuracy in data annotation.
2. Integration with Augmented Reality
Bounding box technology is expected to integrate more with augmented reality (AR) applications. As AR technology matures, the knowledge gained from bounding box implementations will help in object recognition within AR environments, enriching user experiences.
3. Cross-Industry Adaptability
The applications of bounding box technology will transcend beyond traditional sectors, finding new uses in areas such as agriculture, environmental monitoring, and smart cities. This versatility ensures that bounding boxes will remain relevant as industries continue to innovate.
Conclusion
In conclusion, the concept of bounding boxes is indispensable in today’s tech-driven business landscape. Their role in data annotation, machine learning, and practical applications across various industries cannot be overstated. By embracing bounding box technology, organizations can enhance the accuracy of their data processes, optimize operational efficiencies, and stay ahead in an increasingly competitive market.
For businesses like Keylabs.ai, adopting robust bounding box methodologies is not just an operational necessity; it’s a strategic advantage. As technology continues to evolve, those who effectively harness the power of bounding boxes will undoubtedly lead the way in innovation and success.